Do we understand AI through scientific discovery or social decisions?
Part two of my conversation with cognitive scientist Sean Trott
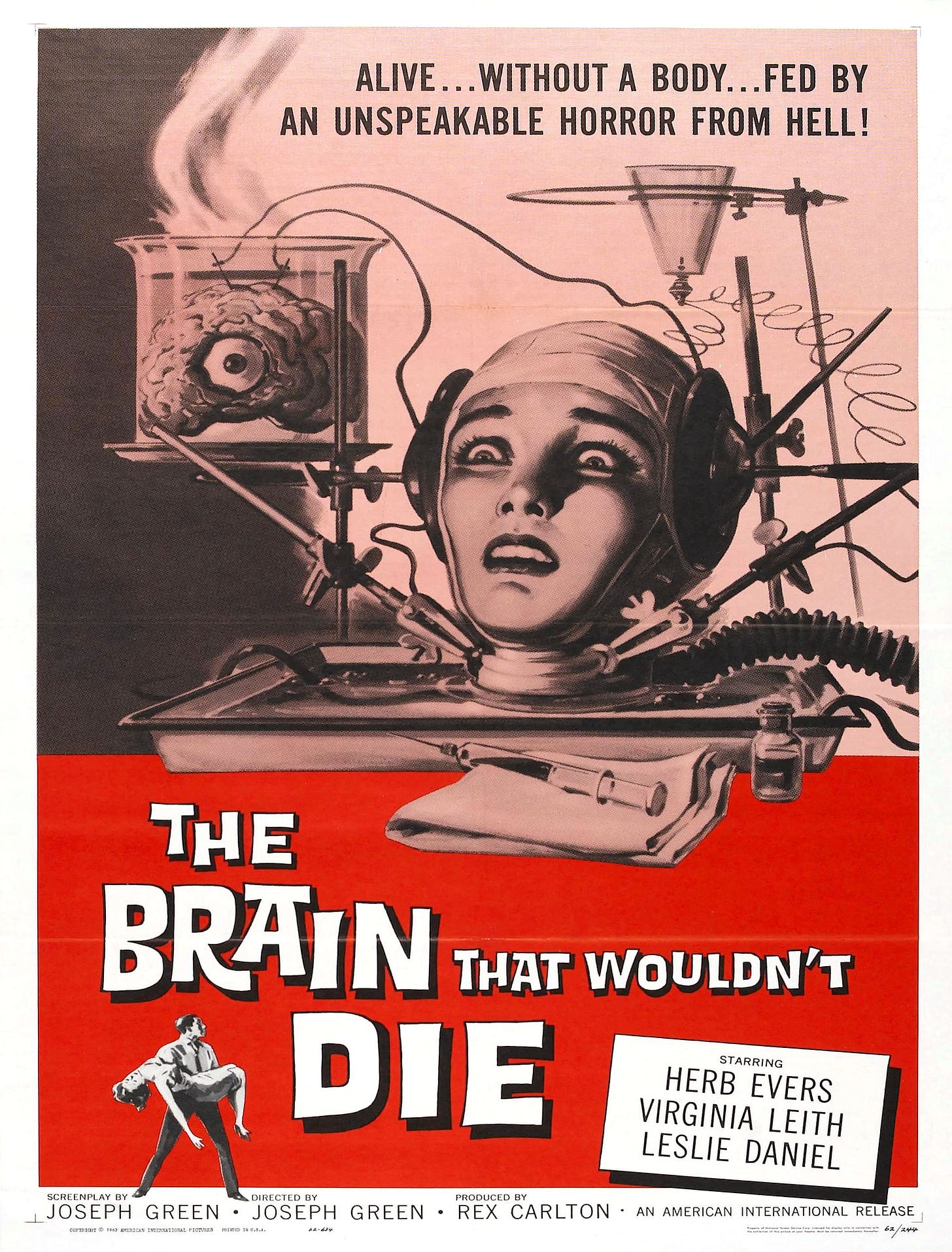
Sean Trott is a cognitive scientist at UC San Diego who studies human cognition and generative AI, topics he covers in delightful detail via his newsletter The Counterfactual, which I highly recommend. A few months ago we began a long conversation over about the scientific study of human minds and large-language models, a conversation that will be shared here on Cognitive Resonance over the next few weeks. In part one, we examined whether we need a new scientific paradigm to understand AI. Here, we turn to the question of how to evaluate AI capabilities and if they are intelligent — and whether the answer is grounded in empirical analysis or social convention. Yes, this post is a little wonky, but it’s worth it.
Ben
Sean, I’ve sensed you are a little frustrated by relying on individual tests of LLMs to determine their capabilities. We might call this the challenge of benchmarking. We are developing various measures to determine whether LLMs are exhibiting some form of what we might describe as consciousness, or sentience, or "artificial general intelligence," etc. But we are doing this in piecemeal fashion, and you find this unsatisfying absent a "theory of generalization."
What I've been wondering lately is whether the entire benchmarking project is itself a bit misguided. At least since Turing and his famous test, we've yearned for some measure to help us determine whether something artificially created should be considered intelligent. This approach presupposes there's an empirical answer to this question – but maybe that's wrong.
I've been thinking a lot about a prescient – if somewhat dense – essay that the philosopher Hilary Putnam wrote nearly 60 years ago, wherein he argued that whether robots (read today: LLMs) are "conscious" will not be the product of discovery but rather decision. Under this view, what ultimately matters is not whether we can devise a test that resolves whether LLMs are intelligent they way we humans are, but instead whether we decide to treat them as "fellow members of our shared linguistic community, or to not so treat them."
If Putnam's on to something here, it suggests cognitive science may be of limited use in helping us understand LLMs. Instead, what will matter most is social practice, how we humans decide how to treat our new "digital friends" or co-pilots or whatever metaphor we end up using.
There are times when it feels like this is happening already. The output of LLMs are sufficiently human-like that people feel as if they are conversing with an intelligent being. This smacks of anthropomorphism but maybe, per Putnam, that charge is less of a counterargument than it is evidence that we are expanding the scope of our "linguistic community" to include these new digital creations. I've had numerous interactions on social media with people who are convinced that LLMs are reasoning, and that they are more than just statistical word-prediction systems.
Perhaps I'm wrong for thinking – perhaps wanting – to exclude them from our human conversation?
Sean
On the topic of testing LLMs, I have a basic complaint: Much of the discourse around what they can or can't do revolves around screenshots or excerpts from what's essentially a single trial ("here is what GPT-4 did when I asked it X...clearly, it is [very intelligent or not]"). Now, a single trial can in some cases be informative: it's useful as an existence proof, say, or for disconfirming an extremely strong null hypothesis that claims some behavior will never be observed.
But there's a reason why in cognitive science, experimenters often run experiments with multiple items. We want to make sure that the behavior we're interested in generalizes across a larger set of scenarios that have been designed using some systematic manipulation. Similarly, most tests of a capacity in humans have more than a single question, and have been designed with at least a little care to tackle the construct that's being investigated.
So at minimum, when there are debates about what LLMs can and can't do, I'd like to see:
A clearer articulation of what capacity is being investigated. What's actually at stake here?
An explanation of why the measure being used to assess that capacity makes sense. What else could we have used and why is this one appropriate?
An explanation of why the evidence being marshaled to make the ultimate claim actually supports that claim. If the system had displayed different behavior, would we have reached a different conclusion?
In short, I'm looking for a more rigorous empirical approach. If we're going to do things empirically, we should do them right. To draw a connection to another domain I know you care a lot about: quantifying and comparing the success of different educational approaches or interventions can be really challenging, and the quality of the evidence is sometimes dim, but it's better to make our way with a dim light than no light at all.
Alternatively, if we're going to forsake empirics altogether (what I've called the "axiomatic rejection" view), we should be clear that this is what we're doing.
This is different from your point about doing tests in a piecemeal fashion. But there's also an interesting question whether a bunch of different tests add up to something meaningful. I'm not really sure, myself—especially when it comes to something like "artificial general intelligence" (AGI). I've generally supported the use of testing to evaluate specific capacities (like "theory of mind"). This is useful theoretically and also (hopefully) for predicting what a system can or can't do.
But AGI feels much fuzzier than that, which is why I mostly haven't written about it or tried to argue systems have it or don't have it. I'm just genuinely not sure what criteria we should be using, and it feels like the term is slippery enough such that people of various persuasions use it to mean different things depending on the goal. Let alone something even harder to define and operationalize like "consciousness"!
Which also speaks to your question – and maybe Putnam's argument – that perhaps questions about consciousness are better conceived as decisions rather than discoveries. I think this is very interesting! And probably true on some level. Though I'd still argue that these decisions are themselves based on empirical data in some sense, e.g., having observed that a robot consistently produces sensible linguistic output in the appropriate situation. It's just that these empirical data aren't really discrete "tests" per se.
Another analogy might be helpful here: should we care about non-human animals—and how much? My personal view is that we should care a lot, and that we should try to minimize their suffering. Now, I have no way of knowing that a cow or a cat feels pain. But if an animal displays the kind of behavior I associate with that capacity—e.g., running away from things I suppose would hurt it—it seems like good evidence to me. Some people would point to further evidence: if that animal also has a similar nervous system, it suggests that the animal is capable of having at least some of the same experiences we do. Of course, considering animals as moral patients or moral agents opens up some even weirder, harder questions (do they have "rights"? what responsibilities do we have towards them?). But my point here is simply that this is another case where ultimately we have to make some kind of decision (and that decision gets negotiated by a bunch of different stakeholders, sometimes contentiously), but that decision also depends on our own experiences or "data".
I'm just not ready to abandon something like empiricism when it comes to understanding these things.
If you are enjoying reading this newsletter, please help spread the word by sharing on social media and on Substack (something I’m still learning how to do)